Unraveling impacts of polycrystalline microstructures on ionic conductivity of ceramic electrolytes by computational homogenization and machine learning
New Publication in “Journal of Applied Physics”
2024/09/09
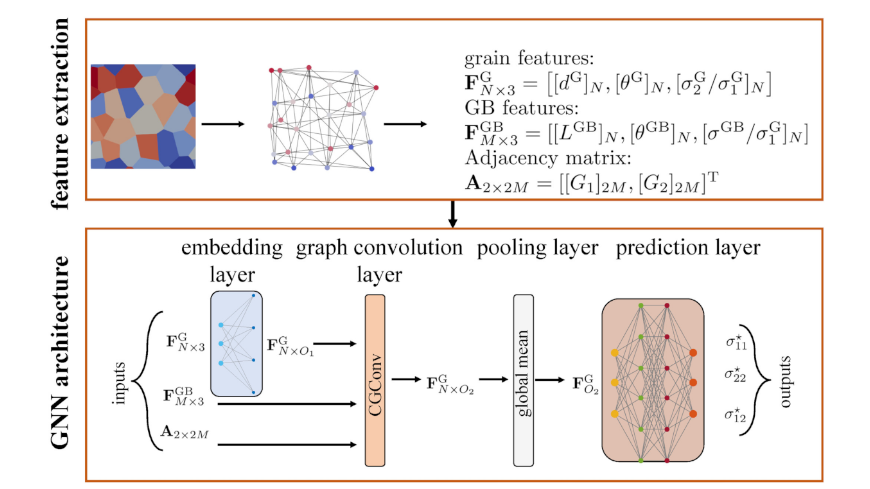
Authors: Xiang-Long Peng, Bai-Xiang Xu
The ionic conductivity at the grain boundaries (GBs) in oxide ceramics is typically several orders of magnitude lower than that within the grain interior. This detrimental GB effect is the main bottleneck for designing high-performance ceramic electrolytes intended for use in solid-state lithium-ion batteries, fuel cells, and electrolyzer cells. The macroscopic ionic conductivity in oxide ceramics is essentially governed by the underlying polycrystalline microstructures where GBs and grain morphology go hand in hand. This provides the possibility to enhance the ion conductivity by microstructure engineering. To this end, a thorough understanding of microstructure–property correlation is highly desirable. In this work, we investigate numerous polycrystalline microstructure samples with varying grain and grain boundary features. Their macroscopic ionic conductivities are numerically evaluated by the finite element homogenization method, whereby the GB resistance is explicitly regarded. The influence of different microstructural features on the effective ionic conductivity is systematically studied. The microstructure–property relationships are revealed. Additionally, a graph neural network-based machine learning model is constructed and trained. It can accurately predict the effective ionic conductivity for a given polycrystalline microstructure. This work provides crucial quantitative guidelines for optimizing the ionic conducting performance of oxide ceramics by tailoring microstructures.
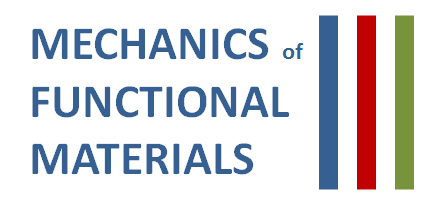