A Machine Learning Enabled Image-data-driven End-to-end Mechanical Field Predictor For Dual-Phase Steel
New Publication in “Proceedings in Applied Mathematics & Mechanics”
2023/03/27
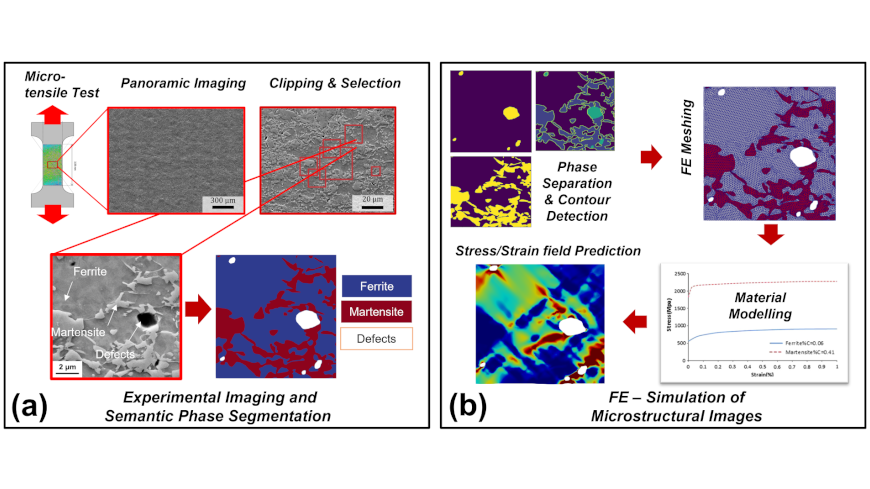
Authors: Binbin Lin, Setareh Medghalchi, Sandra Korte-Kerzel and Bai-Xiang Xu
This contribution presents convolutional neural nets (CNN) based surrogate models for prediction of von Mises stress and equivalent plastic strain fields of commonly used Dual-Phase (DP) steels in automotive applications. The models predict field quantities in an end-to-end manner, driven by segmented phase images from real experimental scanning electron micrographs as inputs and FEM calculations as outputs. Hereby, we train CNN models with the U-net neural network structure based on around 900 elastoplastic FEM simulations of various DP steel microstructure samples under tensile test. The trained CNN models are validated and tested on 250 and 50 samples, respectively. Thereby CNN models are employed sequentially for different tasks , from the real micrographs to segmented phase maps, then from segmented phase maps to stress, strain field predictions, in an end-to-end manner. The field predictor model results show good agreement with the test data and convincing performance on unseen microstructural dataset. This work demonstrates the large potential of a Machine Learning model to make accumulatively use of the physics-based simulation data of large number of boundary value problems with varying microstructure. It recaptures not only the physics, implied in each simulation training data obtained from the partial different governing equations of mechanics, but also the overarching correlation between the microstructure and the stress and strain field responses.
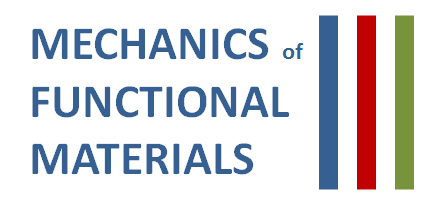