Data-driven microstructure sensitivity study of fibrous paper materials
New Publication in “Materials and Design”
2021/09/30
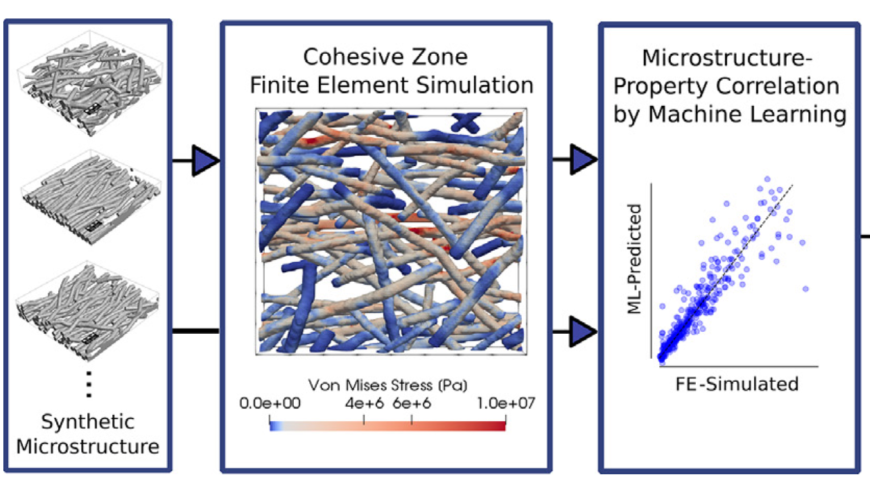
Authors: Binbin Lin, Yang Bai and Bai-Xiang Xu
Nowadays, Machine Learning (ML) model of the structure-property relation based on large data from reliable physical models becomes a new and promising approach for material design. The present work demonstrates such approach to examine the variation in microstructure features on mechanical properties of paper materials. After the generation of a “big” dataset of fiber network samples, morphological feature data, including interfiber contact properties were extracted and statistically evaluated. By performing cohesive finite element simulations, the mechanical properties including failure strain, effective stiffness, and maximal stress of fiber networks under tensile test were determined and served along with structural feature data for the ML analysis. Gradient Boosting method achieved a performance score of approx. 0.9 for all mechanical properties of such complex fibrous struc- ture. It was found that “disorderness” represented by the variation of fiber network orientation and the mean contact area size to be the most influential factors to the failure strain and effective stiffness. Whereas the failure strength was driven by the homogeneous distribution of the contact areas. The results validated the strong ori- entation dependence of fibrous materials in experimental observations and enlighten the importance of sensitiv- ity as feature parameters and the striking potential of ML for material optimization.
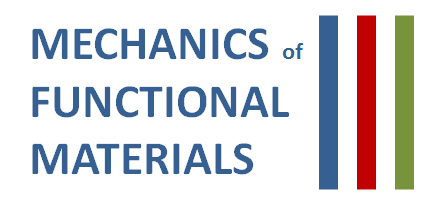